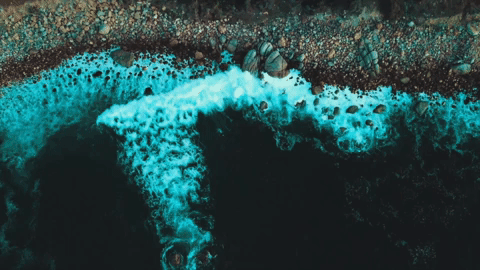
We adopt innovations from the systemic sciences, to enhance educational and learning experiences. It is an evolutionary approach to learning, that offers direct value across both our content and our platform:
(1) Curated expert content8 - critically curated and referenced scientific material;
(2) Platform design using a range of recall and memorisation enhancement factors, like: Colours, Gestalt Design7, Curve of Forgetfulness2, Cognitive shortcuts6, Timed- breaks3, Rich-pictures1, and Rewards.
These twin value propositions are unique features that provide superior and efficient absorption of subject matter. It empowers the entire stakeholder community, be it institutions, individuals and learners.
We are proud to offer an evolutionary type platform to assist vital user community needs, offering enhanced learning tools that includes: Repetition, Dynamic scheduling, Timed lags, Leaner responses, all of which enable better personalised learning.
Importantly, by self-adjusting to unique learner responses, the platform allow for custom lesson delivery, to suit each user. This amplifies study outcomes, and represents a transformative learning method, mimicking cognitive cues that shape our neural pathways. In this way we intend to assist entrepreneurs, professionals, and post-graduates to access the best thinking, the best tools and the best methodologies to resolve real-world problems. We distance ourselves from classical Learner Management Systems (LMS) thinking and design, towards an embodied learner-experience platform, supporting both pedagogy and andragogy. It encourages the holism of andragogy, as it departs from mechanical modes of learning. We design flexibility, inter-leaving, natural and fluid user experiences, endorsed under Social-Emotional-Learning (SEL).
The potential misinterpretations and unreliability of our sensory perceptions (e.g. hearing, sight, touch), dictates learning tools to compliment these deficits, as far as possible. Our platform is designed for scientific reliability and trust, allowing us to share Intellectual Property to support communities of practice in an efficient and quality-rich landscape offering some of the following built-in values:
- Cognitive biases – humans have a few cognitive biases, that may be gainfully employed to enhance recall and memorisation;
- Scheduling and repetition to counter the “forgetfulness curve”;
- Neural pathway widening and densification (new pathways encouraged via trans-disciplinary connections);
- Pattern-seeking – employing rich-picture rapid learning in design of platform and content.
The Value of the Cognitive-Engine
Research confirms personal instruction yields superior results (Bloom, 1984), but since personal instruction has become very expensive, and tends to be inefficient for scaling that are demanded by modern society, we opted to design the Cognitive-Engine to meet these demands. Core attributes of personalised learning is infused into our delivery engine, ranging from instant feedback, reinforcement, reminder settings, reward dashboards all of which serve to imitate a personal teacher. Our self-learning and self-assessment innovations are key milestones in moving toward andragogy, as it removes the anxiety of pedagogical duality.
Adaptive algorithms of the Cognitive-Engine?
By accurately ranking scores or answers, learners produce unique patterns, that generates an adaptive reveal of the cards, thus facilitating active recall and memorisation practices (Brame & Biel, 2015), known to yield long-term retention of new constructs (Karpicke & Blunt, 2011), or conceptual learning.
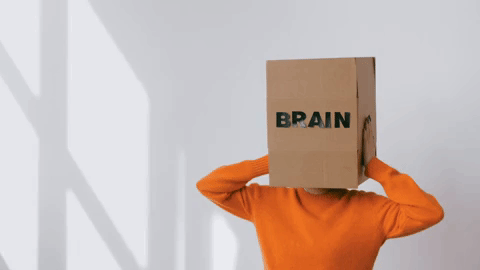
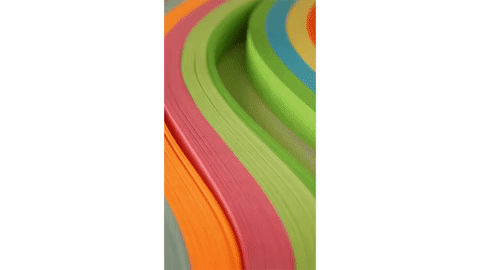
Linear loads of scaffolding and the Cognitive-Engine
A known challenge of passive studying is that material are hard coded into a linear order, which can be tedious, introducing boredom and disinterest since the average number of items we retain in working memory is ”between 5-7” (Miller, 1956). The CE counters this limit, by reshaping concepts originally presented in books, in much more flexible formats, thus focusing upon critical and specific constructs. It allows users to push learning boundaries, beyond current levels of understanding (McLeod, 2014), via inter-leaving (switching between topics), thereby reducing the cognitive load and increasing the desire to continue (Weidman, 2015).
Deepening stimulation and the Cognitive-Engine?
A holistic understanding of complex learning processes promotes learners to create their own cards and decks. The act of condensing data into short bursts of questions and answers engages deeper cognitive processes, to shape these questions and answers. The ability to author, create, edit, and reorder cards and decks to be shared therefore facilitates collaboration. The CE enriches learning by using rich pictures, known to be superior to text based learning alone.
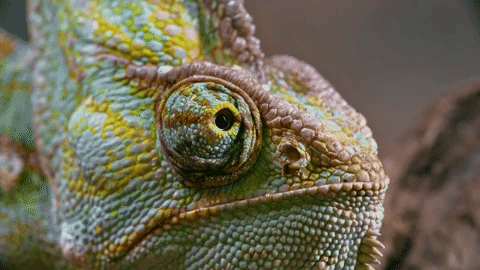
Colour confidence algorithm and the Cognitive-Engine?
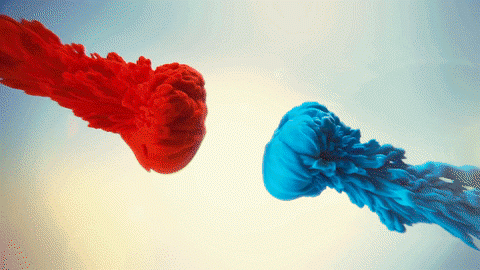
One of our key algorithms prohibits introducing new questions, unless the number of low-confidence cards exceeds a certain value. The closer the learners remain to this value, the more the algorithm repeats these questions until learners upgrade their confidence rating, thus keeping learners in the zone of proximal development. This is coupled to repeating cards having low scores, both of which intensifies reflection and learning. The platform accommodates for over-optimism (scoring high on cards), by virtue of 3 cycles, so as to ensure retention over time, despite error rankings, i.e., re-exposure of data via three cycles entrenches learning and deepens cognitive pathways. Since each rank option are associated with a colour, users choose more accurately over time, as it facilitates authentic self-reflection.
Forgetfulness curve and the Cognitive-Engine?
The repetition system is a vital part of the CE experience, especially since the Forgetfulness Curve (Hermann Ebbinghaus, 1885), reveals the decay of our memory. Typically we retain 100% of what we learn initially, but it steeply drops off since short-term memory is tenuous. It suggests the more or longer we remember something, the less likely we will forget it. In order to reduce the forgetfulness curve, our platform repeat cycles and individual scoring becomes a key strategy to “hack the brain”. We encode into algorithms, our time-based repetition, for long-term memory retention, especially when spaced appropriately (Janiszewski et al., 2003). Another feature is, by allowing the brain to refresh concepts, we lower the forgetting curve effects, as the brain builds neural connections (Schwindel, 2011), highlighting how spaced and timed repetition across knowledge areas yield direct gains (K.S.H, 2016; Roediger III, 2011).
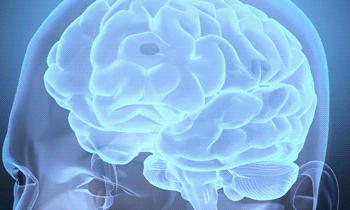